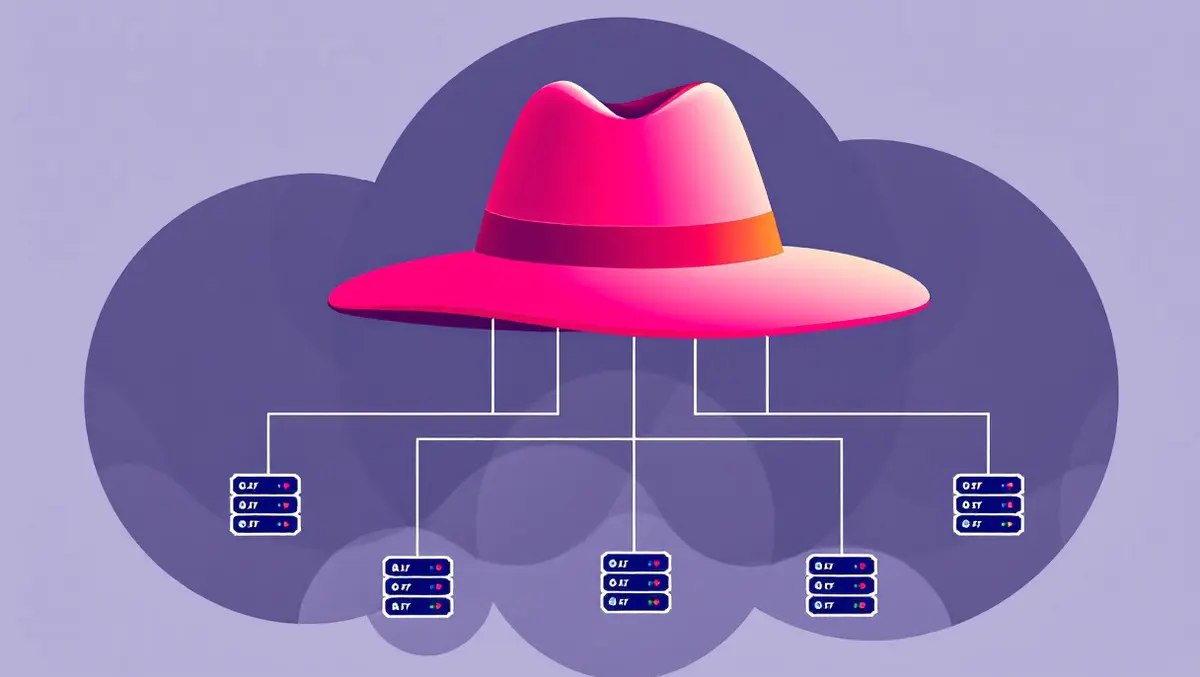
Red Hat leads launch of llm-d to scale generative AI in clouds
Red Hat has introduced llm-d, an open source project aimed at enabling large-scale distributed generative AI inference across hybrid cloud environments.
The llm-d initiative is the result of collaboration between Red Hat and a group of founding contributors comprising CoreWeave, Google Cloud, IBM Research and NVIDIA, with additional support from AMD, Cisco, Hugging Face, Intel, Lambda, Mistral AI, and academic partners from the University of California, Berkeley, and the University of Chicago.
The new project utilises vLLM-based distributed inference, a native Kubernetes architecture, and AI-aware network routing to facilitate robust and scalable AI inference clouds that can meet demanding production service-level objectives. Red Hat asserts that this will support any AI model, on any hardware accelerator, in any cloud environment.
Brian Stevens, Senior Vice President and AI CTO at Red Hat, stated, "The launch of the llm-d community, backed by a vanguard of AI leaders, marks a pivotal moment in addressing the need for scalable gen AI inference, a crucial obstacle that must be overcome to enable broader enterprise AI adoption. By tapping the innovation of vLLM and the proven capabilities of Kubernetes, llm-d paves the way for distributed, scalable and high-performing AI inference across the expanded hybrid cloud, supporting any model, any accelerator, on any cloud environment and helping realize a vision of limitless AI potential."
Addressing the scaling needs of generative AI, Red Hat points to a Gartner forecast that suggests by 2028, more than 80% of data centre workload accelerators will be principally deployed for inference rather than model training. This projected shift highlights the necessity for efficient and scalable inference solutions as AI models become larger and more complex.
The llm-d project's architecture is designed to overcome the practical limitations of centralised AI inference, such as prohibitive costs and latency. Its main features include vLLM for rapid model support, Prefill and Decode Disaggregation for distributing computational workloads, KV Cache Offloading based on LMCache to shift memory loads onto standard storage, and AI-Aware Network Routing for optimised request scheduling. Further, the project supports Google Cloud's Tensor Processing Units and NVIDIA's Inference Xfer Library for high-performance data transfer.
The community formed around llm-d comprises both technology vendors and academic institutions. Each wants to address efficiency, cost, and performance at scale for AI-powered applications. Several of these partners provided statements regarding their involvement and the intended impact of the project.
Ramine Roane, Corporate Vice President, AI Product Management at AMD, said, "AMD is proud to be a founding member of the llm-d community, contributing our expertise in high-performance GPUs to advance AI inference for evolving enterprise AI needs. As organisations navigate the increasing complexity of generative AI to achieve greater scale and efficiency, AMD looks forward to meeting this industry demand through the llm-d project."
Shannon McFarland, Vice President, Cisco Open Source Program Office & Head of Cisco DevNet, remarked, "The llm-d project is an exciting step forward for practical generative AI. llm-d empowers developers to programmatically integrate and scale generative AI inference, unlocking new levels of innovation and efficiency in the modern AI landscape. Cisco is proud to be part of the llm-d community, where we're working together to explore real-world use cases that help organisations apply AI more effectively and efficiently."
Chen Goldberg, Senior Vice President, Engineering, CoreWeave, commented, "CoreWeave is proud to be a founding contributor to the llm-d project and to deepen our long-standing commitment to open source AI. From our early partnership with EleutherAI to our ongoing work advancing inference at scale, we've consistently invested in making powerful AI infrastructure more accessible. We're excited to collaborate with an incredible group of partners and the broader developer community to build a flexible, high-performance inference engine that accelerates innovation and lays the groundwork for open, interoperable AI."
Mark Lohmeyer, Vice President and General Manager, AI & Computing Infrastructure, Google Cloud, stated, "Efficient AI inference is paramount as organisations move to deploying AI at scale and deliver value for their users. As we enter this new age of inference, Google Cloud is proud to build upon our legacy of open source contributions as a founding contributor to the llm-d project. This new community will serve as a critical catalyst for distributed AI inference at scale, helping users realise enhanced workload efficiency with increased optionality for their infrastructure resources."
Jeff Boudier, Head of Product, Hugging Face, said, "We believe every company should be able to build and run their own models. With vLLM leveraging the Hugging Face transformers library as the source of truth for model definitions; a wide diversity of models large and small is available to power text, audio, image and video AI applications. Eight million AI Builders use Hugging Face to collaborate on over two million AI models and datasets openly shared with the global community. We are excited to support the llm-d project to enable developers to take these applications to scale."
Priya Nagpurkar, Vice President, Hybrid Cloud and AI Platform, IBM Research, commented, "At IBM, we believe the next phase of AI is about efficiency and scale. We're focused on unlocking value for enterprises through AI solutions they can deploy effectively. As a founding contributor to llm-d, IBM is proud to be a key part of building a differentiated hardware agnostic distributed AI inference platform. We're looking forward to continued contributions towards the growth and success of this community to transform the future of AI inference."
Bill Pearson, Vice President, Data Center & AI Software Solutions and Ecosystem, Intel, said, "The launch of llm-d will serve as a key inflection point for the industry in driving AI transformation at scale, and Intel is excited to participate as a founding supporter. Intel's involvement with llm-d is the latest milestone in our decades-long collaboration with Red Hat to empower enterprises with open source solutions that they can deploy anywhere, on their platform of choice. We look forward to further extending and building AI innovation through the llm-d community."
Eve Callicoat, Senior Staff Engineer, ML Platform, Lambda, commented, "Inference is where the real-world value of AI is delivered, and llm-d represents a major leap forward. Lambda is proud to support a project that makes state-of-the-art inference accessible, efficient, and open."
Ujval Kapasi, Vice President, Engineering AI Frameworks, NVIDIA, stated, "The llm-d project is an important addition to the open source AI ecosystem and reflects NVIDIA's support for collaboration to drive innovation in generative AI. Scalable, highly performant inference is key to the next wave of generative and agentic AI. We're working with Red Hat and other supporting partners to foster llm-d community engagement and industry adoption, helping accelerate llm-d with innovations from NVIDIA Dynamo such as NIXL."
Ion Stoica, Professor and Director of Sky Computing Lab, University of California, Berkeley, remarked, "We are pleased to see Red Hat build upon the established success of vLLM, which originated in our lab to help address the speed and memory challenges that come with running large AI models. Open source projects like vLLM, and now llm-d anchored in vLLM, are at the frontier of AI innovation tackling the most demanding AI inference requirements and moving the needle for the industry at large."
Junchen Jiang, Professor at the LMCache Lab, University of Chicago, added, "Distributed KV cache optimisations, such as offloading, compression, and blending, have been a key focus of our lab, and we are excited to see llm-d leveraging LMCache as a core component to reduce time to first token as well as improve throughput, particularly in long-context inference."