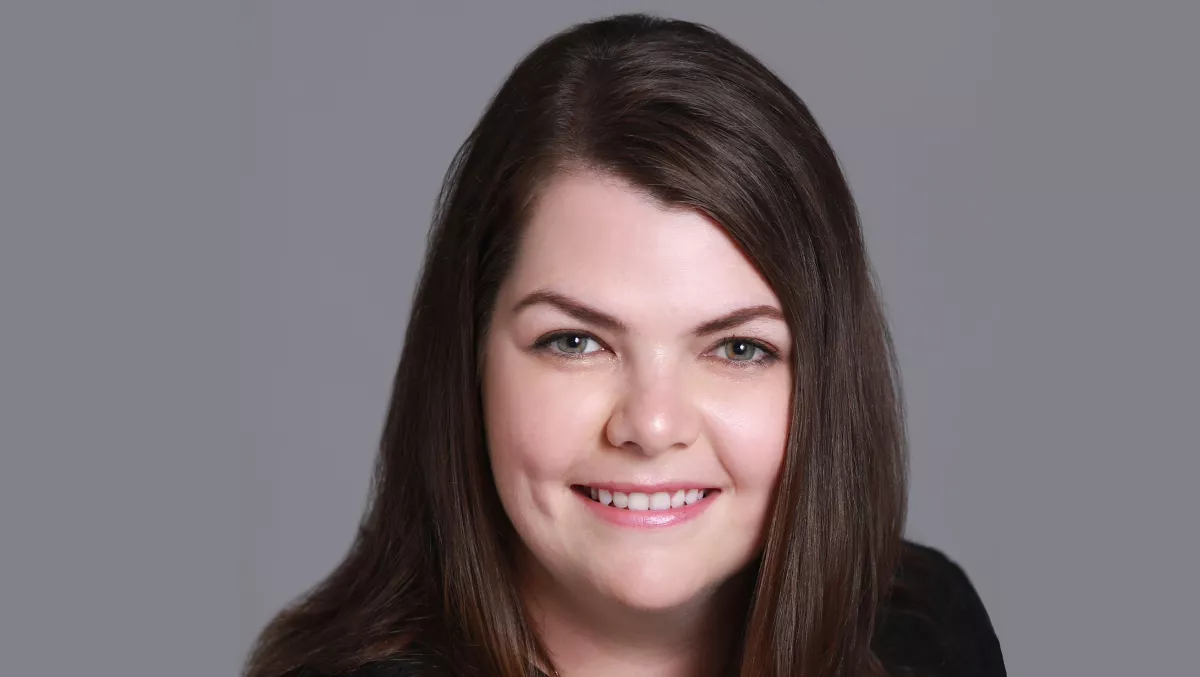
Q&A: Cloudera on building the perfect hybrid cloud architecture
Cloudera's APJ cloud director Stevie Walsh chats to us about hybrid cloud and the democratisation of data.
One of the most common pieces of advice to organisations is that an entirely on-prem IT infrastructure is seldom workable for most organisations' IT needs, so the hybrid infrastructure is the best of both worlds. Is there a trick (or perhaps several tricks) to making the most of all these environments?
Many organisations take a well-intentioned leap into a hybrid cloud environment without taking the entire digital transformation journey into account - essentially putting the cart before the horse.
They're on the cloud, but their data could be spread across multiple clouds. There are also situations where they must split their data between the cloud and on-premise, which makes it even more challenging to access, manage and secure.
All clouds have their benefits, however, there are common challenges that need to be addressed to make the most of today's modern cloud environments. These include:
- Greater amounts of data coming from multiple sources
- Data capture at the Edge, where it must be ingested and analysed in real-time
- Multiple systems that can be problematic to integrate
- Concerns related to data security and accessibility of data within an organisation
- Multiple public and provide clouds and data warehouses within a single enterprise customer
It's also important to understand which workloads are best suited for each deployment – the public cloud is ideal for intermittent, seasonal, 'batchy' or unknown (newly developed workloads) whereas a private cloud is best for highly utilised workloads. Hybrid cloud environments can help organisations support increasingly remote workforces, improving scalability and control while maintaining business continuity.
Having a single platform for both public and private provides organisations with the possibility to choose the right destination for workloads or launch, develop or sandbox new workloads in the cloud and then move them on-prem.
In many cases, data needs to be used by both analysts and less technical users, as well as machine learning or automated systems. Does data need to be 're-engineered to cater for all these different users and applications?
A decentralised structure allows for greater productivity benefits from data and analytics efforts. Customers use data to accelerate innovation efforts by combining existing technologies in new ways, or "upcycling" existing technologies by applying them to a different domain to solve a different problem.
Data democratisation is the idea of empowering every employee (not just the data scientists) with the training and tools to be data-savvy. Compared to the IT team, business user groups like HR, marketing or product development have a more in-depth understanding of the data collection context and will be able to come up with domain-specific actionable insights.
For example, the marketing team in a financial services organisation may need data modelling to develop a recommendation engine for lead capture. Within the same company, the customer support team might be on using data modelling to understand and reduce customer churn.
The roadblock, however, is often legacy data systems that don't have the high-speed processing power to support the instantaneous delivery of ensuring the right data reaches the right people at the right time.
Applying Machine learning (ML) is another area of significant untapped potential – businesses that have adopted a successful ML strategy are outperforming their competitors by over 9%.
To help make it faster and simpler for businesses to unlock greater ML capabilities, we recently released Applied ML Prototypes (AMPs). Everyone is trying to do more with their data and AMPs enable data teams to go from idea to a fully working ML use case in a fraction of the time.
Enterprise data clouds help organisations use analytics at every stage of the data lifecycle, working at speed to extract the true value from their data. They also enable users to control who has access to data in a secure manner that maintains data integrity.
Speaking broadly about the democratisation of data, what does this mean in a general enterprise/business context?
With data playing an increasingly strategic role across all industries, data democratisation is something that should no longer be considered optional. Providing employees with access to data ensures they can gain better insights to make informed decisions quickly. Benefits can come in the form of financial or operational improvements such as increased operational efficiencies, new revenue streams, increasing average spend per customer, resilience and cost savings. To get started on data democracy, businesses need to look at structure, tools and training.
One of the barriers to data democracy is, ironically, organisational structure. It is common for businesses to have centralised data analyst teams. It is also common for data to be kept in disparate silos, and data teams need time to extract and consolidate the relevant data. Such structures can often delay the decision-making process. Removing these data silos ensure more people can access the data, empowering them to make smarter, data-driven decisions.
As businesses push data and analytics out to more people within their organisation, it's important they manage risk around sensitive information and also consider how to remain compliant with governmental regulations related to data privacy and locality. There are various strategies to achieve these goals, but the key is simplifying the security and governance end to end.
Being able to deliver consistent security and governance across all clouds - public or private rather than multiple security solutions stitched together reduces operational complexity, risk, and ongoing costs.
How can data democratisation be applied to different industry sectors?
Locally, within ANZ, telco providers are leveraging customer experience analytics to build a 360-degree view of their customer journey across all channels and lines of business. From this, they're building prediction models to identify a customer base for personalised offers, even during this uncertain time.
When it comes to the in-person retail experience, technology and widespread access to data is proving a game-changer. Businesses can now combine the power of connected technology with a physical presence, placing beacons and sensors in stores to track purchase paths of customers by identifying 'hot' and 'cold' spots throughout the store. In addition, they can utilise facial recognition technologies to measure customer moods and sentiments.
On the supply chain front, retailers can use IoT devices to track goods, for instance, embedding devices within refrigerated trucks carrying perishable foods to measure temperature and humidity.
There are times when organisations need timely and accurate data to make quick decisions, such as in emergency response situations. How does data democratisation fit into these situations and these decision-making processes?
In an emergency response situation, the need for speed is mission-critical, to make sense of data accurately and quickly. Organisations need to be able to ingest and process data in real-time. Decisions can then be made faster and with confidence, often as a situation is unfolding.
For example, to mitigate the impacts of power outages, utility companies are now using advanced data analytics and machine learning to monitor and manage capacity, build predictive capacity models, identify bottlenecks, and prioritise and plan network expansion decisions. They're also using advanced analytics to predict network capacity, mitigate business disruption and effectively 'keep the lights on'.
Another of our Australian based customers is in the financial services industry. For them, having a single pane of glass view across all their data is critical to support their fraud detection programme, mitigating risk for themselves and their customers. Existing in-house tools were inadequate in managing their data workloads considering the increasing scale of data clusters and demand. The company harnessed our platform to process large volumes of data in real-time and deliver analytical insights on transactions for reporting. This in turn enabled the customer to detect issues and irregularities, proactively avoiding fraudulent transactions.
Is there anything else you would like to add?
Data is a strategic asset that warrants its own strategy in the form of an enterprise data strategy. It's very easy for organisations to lose sight of their initial motivation of migrating to the cloud. What I hear all too often is, "we're going cloud – why are we doing this again?". Sticking to strategy, or being able to steer the ship as needed, is critical especially when it comes to scaling data and ensuring strong governance and security.
Equally important is separating data strategy from cloud and infrastructure strategy to give customers choice and flexibility. Delivering all these elements relies on the right set of tools to start with, consideration of end-to-end data lifecycle and building complex data pipelines with a single platform.