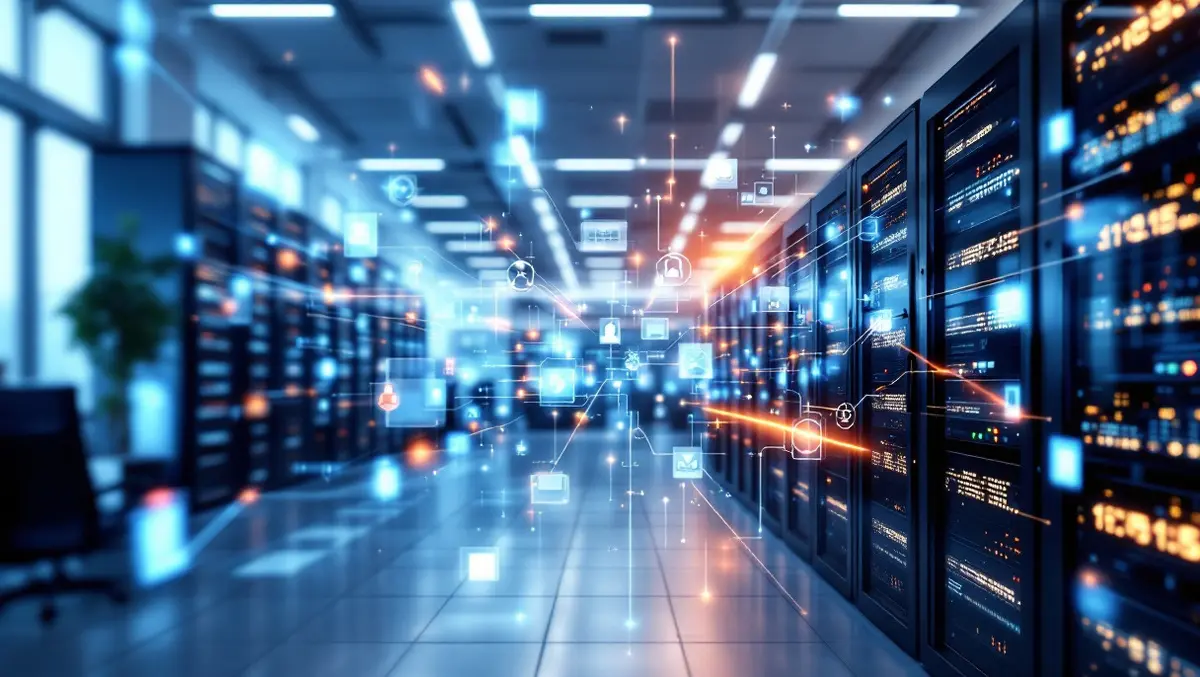
Edge AI deployment enables enterprises to save $2.07 million
An economic analysis by Latent AI has identified a shift within enterprise artificial intelligence from cloud-centric models to edge deployments, highlighting significant potential cost savings and scalability improvements for organisations.
The study, entitled "From Cloud-First to Edge-First: The Future of Enterprise AI," presents 2025 as a pivotal year for this transition, citing factors such as rising cloud computing costs, ongoing shortages of GPUs, and increasing energy prices as contributing elements making edge AI deployments more financially attractive.
Latent AI, which focuses on edge AI solutions for national security and defence, conducted the research to investigate both the challenges and the opportunities in adopting edge AI within industries where timely AI adoption is regarded as a key competitive factor.
Jags Kandasamy, Chief Executive Officer and Co-founder of Latent AI, said: "As enterprise leaders scale AI deployments, they must weigh performance gains against infrastructure investments when evaluating edge versus cloud strategies. With tighter budgets and growing demands for real-time processing, organizations can no longer afford the heavy computational costs of cloud-only solutions. This is where edge-optimized AI proves transformative. From Cloud-First to Edge-First explains how an edge-first approach reduces hardware requirements by 92% while preserving model accuracy, enabling broader AI deployment and enhancing competitive advantage."
The analysis assesses both direct costs, such as hardware and energy, and indirect benefits, such as operational continuity, deployment speed, and lifecycle management. To illustrate the economic impact, the report examines a manufacturing company that initially used a cloud-based AI system with 50 GPUs to process 100 image streams for defect detection across its production line.
The company incurred hardware costs of USD $224,000 per site, which rendered expansion across multiple locations impractical due to financial constraints.
Applying edge AI optimisation and advanced quantisation techniques allowed the manufacturer to reduce its GPU requirement from 50 to 4 per site. This represented a 92% reduction, lowering hardware expenditure to USD $18,000 per deployment and saving USD $207,000 per site, or a total of USD $2.07 million if scaled across ten locations.
Along with the decrease in hardware, memory use fell by 73%—from 14.1GB to 3.8GB per model—while inference speed improved by 73%, boosting defect detection time from 55.2 milliseconds to 14.7 milliseconds. The report noted that model accuracy remained nearly identical, with AU-ROC scores of 0.99127 versus 1.0. The efficiencies also extended to energy, with savings between 65% and 80%, and the elimination of network transfer costs as a result of data being processed locally.
The analysis identifies additional factors driving the economic inflection point for edge AI. Among these are optimisation technologies such as mature quantisation and pruning, which enhance edge model performance and reduce resource requirements; AI accelerators designed by companies like NVIDIA that allow powerful AI processing on resource-constrained devices; and the need for reliability in mission-critical deployments, where edge AI can offer resilience and eliminate dependence on cloud infrastructure.
The research also highlights the impact of evolving data privacy regulations, including those resembling GDPR, which increasingly favour local data processing to reduce both data transmission requirements and compliance concerns. More mature development frameworks are now available, which Latent AI says simplifies edge deployment and makes access easier for a broader range of organisations.
Kandasamy commented further: "Technology shifts don't happen overnight. They build momentum until a tipping point emerges. For edge AI, 2025 is widely recognized as that moment, mirroring the rise of cloud computing in the early-to-mid 2000s. We're seeing technological maturity, economic pressures, and market needs align to drive rapid adoption, offering enterprises a rare chance to gain a lasting edge."
The report makes several recommendations for organisations seeking to leverage the cost advantages of edge AI. These include investing in infrastructure tailored to edge deployments, such as servers and IoT devices designed for scalability; optimising AI models for low-latency, energy-efficient performance at the edge; and balancing the use of cloud and edge resources, with a focus on training in the cloud and inference at the edge to reduce ongoing costs.